AI Embeddings: Unveiling the Power of Artificial Intelligence
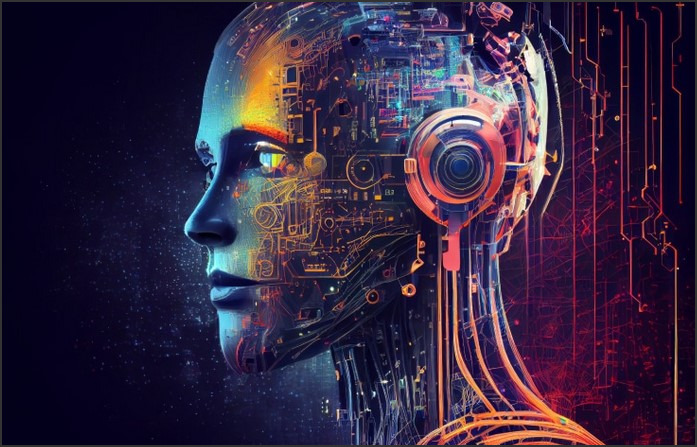
Source : https://cyber-security-speakers.com
AI Embeddings are a powerful tool for Artificial Intelligence (AI) applications. They are a way of representing words, phrases, and other data in a numerical form that can be used by AI algorithms. AI Embeddings are used in a variety of tasks, such as natural language processing, machine translation, and image recognition. They are also used to improve the accuracy of AI models and to reduce the amount of data needed to train them. AI Embeddings are an important part of the AI revolution, and they are helping to unlock the power of AI.
Exploring the Benefits of AI Embeddings for Natural Language Processing
Artificial Intelligence (AI) embeddings are a powerful tool for Natural Language Processing (NLP) tasks. AI embeddings are vector representations of words, phrases, and other linguistic elements that capture the semantic and syntactic relationships between them. These representations are learned from large corpora of text and can be used to improve the accuracy of NLP tasks such as sentiment analysis, text classification, and machine translation.
The use of AI embeddings has been shown to improve the performance of NLP tasks by providing a more accurate representation of the meaning of words and phrases. AI embeddings capture the context of words and phrases, allowing for more accurate predictions of the sentiment or intent of a given text. For example, AI embeddings can be used to distinguish between words with similar meanings but different connotations, such as “happy” and “glad”. AI embeddings can also capture the relationships between words, allowing for more accurate predictions of the sentiment or intent of a given text.
AI embeddings can also be used to improve the accuracy of text classification tasks. By capturing the semantic and syntactic relationships between words, AI embeddings can be used to accurately classify text into different categories. For example, AI embeddings can be used to classify text into topics such as politics, sports, or entertainment. AI embeddings can also be used to identify the sentiment of a given text, allowing for more accurate predictions of the sentiment or intent of a given text.
Finally, AI embeddings can be used to improve the accuracy of machine translation tasks. By capturing the semantic and syntactic relationships between words, AI embeddings can be used to accurately translate text from one language to another. This can be especially useful for translating text from one language to another with different grammatical structures, such as English to Spanish.
In conclusion, AI embeddings are a powerful tool for Natural Language Processing tasks. AI embeddings can be used to improve the accuracy of sentiment analysis, text classification, and machine translation tasks. AI embeddings capture the semantic and syntactic relationships between words, allowing for more accurate predictions of the sentiment or intent of a given text. AI embeddings can also be used to accurately classify text into different categories and to accurately translate text from one language to another.
How AI Embeddings are Revolutionizing Machine Learning Algorithms
Artificial intelligence (AI) embeddings are revolutionizing the way machine learning algorithms are used to solve complex problems. Embeddings are a type of representation that maps data points to a continuous vector space, allowing for more efficient and accurate analysis of data. By using embeddings, machine learning algorithms can better understand the relationships between data points and make more accurate predictions.
Embeddings are particularly useful for natural language processing (NLP) tasks, such as sentiment analysis and text classification. By mapping words to a continuous vector space, embeddings can capture the semantic relationships between words and phrases. This allows machine learning algorithms to better understand the context of a sentence and make more accurate predictions. For example, embeddings can be used to identify the sentiment of a sentence by mapping words to a vector space that captures the sentiment of the words.
Embeddings are also useful for computer vision tasks, such as object recognition and image classification. By mapping images to a continuous vector space, embeddings can capture the relationships between objects in an image and make more accurate predictions. For example, embeddings can be used to identify the objects in an image by mapping images to a vector space that captures the relationships between objects.
Overall, AI embeddings are revolutionizing the way machine learning algorithms are used to solve complex problems. By mapping data points to a continuous vector space, embeddings can capture the relationships between data points and make more accurate predictions. This has enabled machine learning algorithms to better understand the context of data and make more accurate predictions. As a result, AI embeddings are becoming increasingly popular in the field of machine learning and are expected to continue to revolutionize the field in the future.AI Embeddings have revolutionized the way Artificial Intelligence is used in many applications. By providing a way to represent words and phrases in a numerical form, AI Embeddings have enabled machines to understand the meaning of words and phrases in a much more accurate and efficient way. This has enabled AI to be used in a variety of tasks, from natural language processing to image recognition. AI Embeddings have opened up a world of possibilities for Artificial Intelligence, and it is clear that they will continue to be an important part of the future of AI.